Exploring Discrete Event Dynamics for Operations of Intelligent Systems
- Giulia Pedrielli
- May 16, 2021
- 2 min read
Updated: Jul 14, 2021
01/06/2018 We start Our Journey with Intelligent Manufacturing. Thanks NSF!!!
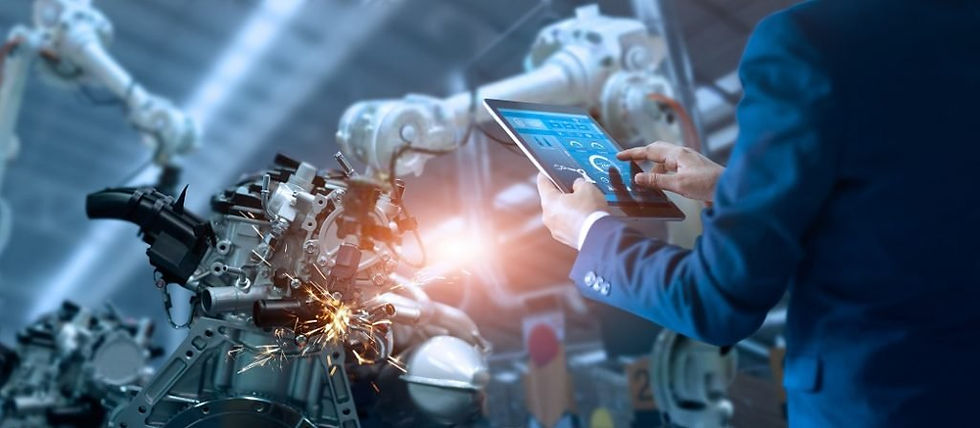
Data, models algorithms are permeating modern factories, but it is still difficult to harmoize data and different available methods to operate complex manufacturing systems. We investigate new models and algoriths for data-driven model informed operation and control of intelligent manufacturing systems.
NSF funded project: EAGER: Exploring Discrete Event Dynamics to Model and Control Intelligent Manufacturing Systems.
This EArly-concept Grant for Exploratory Research (EAGER) project will improve the nation's competitiveness by investigating new models and optimization techniques for control of intelligent manufacturing systems. Rapid advances in computer-controlled processes, high-performance computing, and Internet-of-Things (IoT) lay the groundwork for significantly improving manufacturing productivity. Despite the opportunity, modeling methodologies and real-time control methods continue to face two major challenges, namely the lack of predictive models for the dynamic evolution of manufacturing systems, and the lack of real-time optimization and control algorithms to generate effective on-line production control. This project will address these challenges, which will lead to significant advancement in manufacturing practice. The research is integrated with an education plan to enhance education and outreach activities in underrepresented groups such as Women in Operations Research and Management Science community.
This EAGER award supports fundamental research in methods to control complex manufacturing systems by leveraging both real-time information on machine state and synthetic data generated by in-process simulators. This research will provide new methods for targeted on-demand simulation, integrated with novel control methodology, to support factory level decision-making based on instantaneous machine status. Specifically, a novel simulation and control architecture for intelligent manufacturing systems will address the following main research objectives: (1) construct approximate and high-fidelity simulations that can continuously receive information from the real system and generate conditional statements; and (2) define a class of dynamic performance specifications to be controlled and optimized. This project will lead to aggregate-state models and conditional simulators feeding closed loop predictive controllers that effectively utilize the dynamically changing system state information to dynamically adapt the control policy.
Group: PIs: Pedrielli Giulia (PI), Feng Ju (Co-PI) Students:
(Ph.D.) Kang, Y., Liu, M.
(Master) Gaurav Sharma, Michaela Starkey, Yayati Katkade, Chandrasekar Komaralingam
Results (Selected Publications)
Kang, Y., Mathesen, L., Pedrielli, G., Ju, F. and Lee, L.H., 2020. Multi-fidelity modeling for analysis and optimization of serial production lines. IEEE Transactions on Automatic Control.
Candelieri, A., Pedrielli, G. 2021. Treed-Gaussian Processes with Support Vector Machines as Nodes for Nonstationary Bayesian Optimization. Submitted to INFORMS Winter Simulation Conference 2021. Accepted.
Liu, M., Cao, Y., Pedrielli, G. 2021. Partitioning and Gaussian Processes to Accelerate Sampling in Monte Carlo Tree Search for Continuous Decisions. Submitted to INFORMS Winter Simulation Conference 2021. Accepted.
Pedrielli, G. R. and Barton, R. (2019). Metamodel-Based Quantile Estimation for Hedging Control of Manufacturing Systems. Proceedings of the Winter Simulation Conference. Published. 0891-7736.
Interested in our research?
Reach out to us if you are interested in working in this thematic area!
Comments